
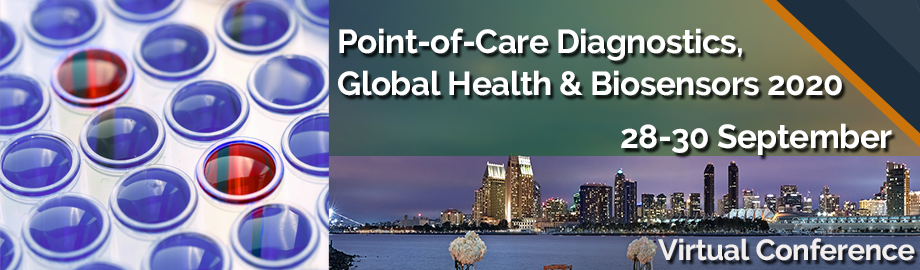
Tan, Multi-agent decision fusion for motor fault diagnosis, Mechanical Systems and Signal Processing 21 (2007) 1285–1299. Chandroth, The “Test and Select” Approach to Ensemble Combination, MCS 2000, LNCS 1857, 30–44. Xu, Fault diagnosis based on support vector machine ensemble, in Proceedings of the Fourth International Conference on Machine Learning and Cybernetics, Guangzhou (2005) 18–21. Zi, Fault diagnosis of rotating machinery based on improved wavelet package transform and SVMs ensemble, Mechanical Systems and Signal Processing 21 (2007) 688–705. Murphey, Case base reasoning in vehicle fault diagnostics, in: Proceedings of the International Joint Conference on Neural Networks 2003, Vols. Sayeed, Model-based reasoning for fault diagnosis of twin spool turbofans, Proceedings of the Institution of Mechanical Engineers, Part G: Journal of Aerospace Engineering 212 (1998) 109–116. Pickles, Expert systems for machine fault diagnosis, Acoustics Australia 22 (1994) 85–90. Pantelides, Random Forests Identification of Gas Turbine Faults, in proceedings of the 19th International Conference on Systems Engineering, (2008) 127–132.Ĭ. Han, Random forests classifier for machine fault diagnosis, Journal of Mechanical Science and Technology 22 (2008) 1716~1725.
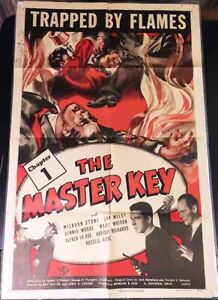
Chu, Support vector machines-based fault diagnosis for turbo-pump rotor, Mechanical Systems and Signal Processing 20 (2006) 939–952.ī. Xu, Fault diagnosis using support vector machine with an application in sheet metal stamping operations, Mechanical Systems and Signal Processing 18 (2004) 143–159. Kordon, Fault diagnosis based on Fisher discriminant analysis and support vector machines, Computers and Chemical Engineering 28 (2004) 1389–1401. Hong, Multiclassification of tool wear with support vector machine by manufacturing loss consideration, International Journal of Machine Tools & Manufacture 44 (2004) 1179–1187. Huang, Fault diagnosis of rotating machinery based on multi-class support vector machines, Journal of Mechanical Science and Technology 19 (2005) 846–859. An, ART-KOHONEN neural network for fault diagnosis of rotating machinery, Mechanical Systems and Signal Processing 18 (2004) 645–657.ī. Samanta, Artificial neural networks and genetic algorithms for gear fault detection, Mechanical Systems and Signal Processing 18 (2004) 1273–1282.ī. Wang, Classification of wavelet map patterns using multi-layer neural networks for gear fault detection, Mechanical Systems and Signal Processing 16 (2002) 695–704.ī.

Nandi, Fault detection using support vector machines and artificial neural networks, augmented by genetic algorithms, Mechanical Systems and Signal Processing 16 (2002) 373–390.ĭ. Al-Balushi, Artificial neural network based fault diagnostics of rolling element bearings using time-domain features, Mechanical Systems and Signal Processing 17 (2003) 317–328. Esat, Artificial neural network based fault diagnostics of rotating machinery using wavelet transforms as a preprocessor, Mechanical Systems and Signal Processing 11 (1997) 751–765.ī. The results obtained were very encouraging and showed that the proposed prognostics system has the potential to be used as an estimation tool for machine remnant life prediction in real life industrial applications.ī.
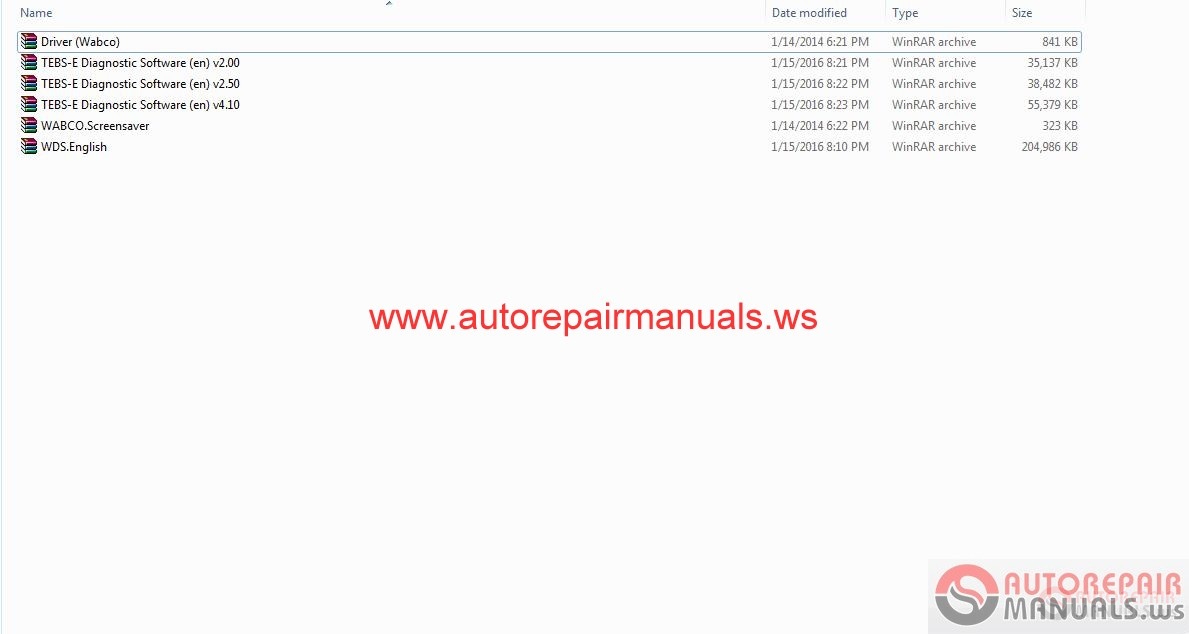
In addition, two sets of impeller-rub data were analysed and employed to predict the remnant life of pump based on estimation of health state probability using the Support Vector Machine (SVM) classifier. To validate the feasibility of the proposed model, the five different level data of typical four faults from High Pressure Liquefied Natural Gas (HP-LNG) pumps were used for the comparison of intelligent diagnostic test using five different classification algorithms.
#INTERNATIONAL MASTER DIAGNOSTICS HP INCREASE SERIES#
To estimate a discrete machine degradation state which can represent the complex nature of machine degradation effectively, the proposed prognostic model employed a classification algorithm which can use a number of damage sensitive features compared to conventional time series analysis techniques for accurate long-term prediction. This paper presents a technique for accurate assessment of the remnant life of machines based on health state probability estimation technique and historical failure knowledge embedded in the closed loop diagnostic and prognostic system. Effective machine fault prognostic technologies can lead to elimination of unscheduled downtime and increase machine useful life and consequently lead to reduction of maintenance costs as well as prevention of human casualties in real engineering asset management.
